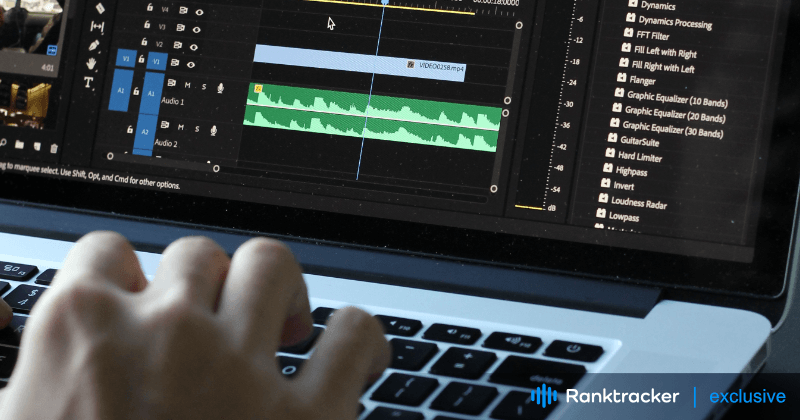
Intro
The role of metadata is crucial in managing data quality as it offers insights into the structure, context, and meaning of datasets. This article will discuss the significance of metadata in maintaining high-quality data and outline strategies for its management.
Exploring Metadata
Metadata serves as information about the data itself, providing administrative details that aid users in understanding and interpreting datasets. Adding context and meaning to data metadata facilitates data discovery, organization, and interpretation. Now, let’s explore the importance and other insights in this data quality management guide.
The Importance of Metadata in Data Quality Control
1. Enhancing Data Understanding: Metadata assists in comprehending the structure, relationships, and characteristics of datasets. Through metadata analysis, organizations can gain insights into their data assets. Make decisions regarding data quality.
2. Ensuring Data Governance: Comprehensive metadata management is essential for data governance. Metadata helps define rules and policies for data usage to ensure compliance with regulations. It also aids organizations in establishing ownership and accountability for their datasets.
3. Facilitating Data Integration: In the integration of datasets from sources metadata plays a vital role in aligning different schemas and definitions. It promotes integration by offering an understanding of the involved data elements.
**4. Discovering Data: **Search capabilities are essential for finding datasets in a vast collection of information within an organization. Metadata plays a role in ensuring search results by defining specific attributes like dataset names, creator details, creation timestamps, and assigned keywords/tags. This makes it simpler for users to locate the information they need quickly.
Effective Metadata Management Strategies
1. Thorough Documentation: It is vital to document metadata at every stage to uphold data quality standards. Make sure that important details such as dataset names, descriptions, and identification codes are well documented for reference.
2. Organized Taxonomy: Creating a taxonomy system helps in categorizing datasets based on characteristics or attributes. This enables filtering and grouping of datasets during different data management phases.
3. Understanding Data Lineage: Establishing data lineage is key to maintaining data quality over time. By tracking the origins, transformations, and movements of data, organizations can identify any anomalies or errors and address them promptly.
The All-in-One Platform for Effective SEO
Behind every successful business is a strong SEO campaign. But with countless optimization tools and techniques out there to choose from, it can be hard to know where to start. Well, fear no more, cause I've got just the thing to help. Presenting the Ranktracker all-in-one platform for effective SEO
We have finally opened registration to Ranktracker absolutely free!
Create a free accountOr Sign in using your credentials
4. Automation Assistance: Leveraging metadata enterprise quality management software that automate the extraction, storage, and sharing of metadata can streamline the process by minimizing tasks while ensuring consistency and accuracy.
5. Foster Collaboration: Encourage collaboration among teams involved in managing metadata processes to enhance efficiency. Different perspectives from a variety of stakeholders can bring insights that enhance the quality and usability of metadata.
6. Reviewing Metadata Processes: It is important to review and validate existing metadata to maintain relevance and accuracy. Conducting audits can help identify any inconsistencies or gaps in the metadata repository and address them proactively.
Challenges in Managing Metadata
Although metadata has advantages, effectively managing it can pose challenges for organizations:
1. Setting Standards: Establishing formats, tagging methods, and naming conventions for metadata requires planning to ensure consistency across different datasets.
2. Ensuring Compliance: Adhering to evolving regulations on data governance practices adds complexity to maintaining up-to-date metadata records.
3. Addressing Interoperability: Integrating systems or platforms while preserving contextual information may be difficult due to differences in formats or semantics used by each application.
Approaches to Maintaining Metadata Accuracy
1. Data Profiling: Data profiling involves analyzing the quality, accuracy, and completeness of metadata. Regularly conducting data profiling helps organizations identify any inconsistencies or errors in their metadata that need attention.
2. Implementing Data Validation Checks: Establishing data validation checks is crucial for ensuring that metadata remains accurate and reliable over time. One way to ensure data accuracy is by checking data formats, maintaining integrity rules, and ensuring data consistency across datasets.
3. Reviewing Metadata Quality: Establish a system for conducting reviews of metadata quality. This includes assessing the significance, correctness, and completeness of metadata attributes based on predefined criteria or guidelines. Any discrepancies or variations identified during these evaluations should be promptly addressed.
Summary
Metadata plays a role in managing data quality as it provides insights into the structure, context, and interpretation of datasets. By managing metadata through documentation, organized taxonomy systems, automation tools, collaboration among stakeholders, and routine audits/reviews. Organizations can unlock the full potential of their datasets while ensuring top-notch information at all levels.